In response to this reality, companies like Google are proposing and researching voice-to-text technology that can accurately capture and transcribe medically relevant information. At the same time, medical technology companies like Tempus are creating artificial intelligence that analyzes medical data and helps providers deliver precision care.
Together, it’s believed that medical voice technology and artificial intelligence can liberate medical care providers from an immense documentation burden.
How Does AI’s Voice-to-Text Technology Assist Medical Providers?
So how does AI transcription software work? Here’s an ideal scenario.
As patient and provider chat, their conversation is being recorded by a mindfully placed, high-quality microphone connected to a computer connected to the internet. Everything is being collected consensually. The patient’s privacy is secure and they can opt-out at any time. As the provider and patient flow through the visit, medical AI:
- Transcribes the conversation
- Analyzes what’s being said for medical relevance
- Cross-references the conversation with the patient’s holistic medical history
- Cross-references the conversation against all available medical data, research, and best practices
- Reports its findings
- Makes diagnosis and treatment recommendations customized to the patient based on findings
- Curates relevant parts of the conversation for documentation.
- Sorts the curated pieces of conversation into topics (e.g., present complaint, social history, family history, drug history)
- Identifies the medical coding needed to eventually acquire payment
At the close of the visit, the provider adds any data they feel is relevant and any data the AI can’t glean from audio recordings such as non-verbal cues. The AI makes recommendations, but the provider always makes the final decision regarding what to propose to the patient. The voice technology captures the final decision, and the AI auto-generates a document for the medical provider to review for accuracy.
Keep reading to learn how the current state of voice-to-text and AI measure up to this model situation.
The Benefits and Promises of Medical Voice Technology
Privacy is a central concern in medical voice technology development.
While voice technology for consumers is currently riddled with privacy issues, patient privacy seems to consistently be at the center of conversations in medical voice technology (MVT) development.
With the Health Information Portability and Accountability Act (HIPAA) going strong since 1996, concern for patient privacy has now become an embedded part of medical culture. In the many conversations regarding the promise of MVT, questions about privacy are central. Researchers and tech developers are thinking about things like how to keep MVT on only when cued, and how to censor sensitive output information when a patient has brought a support person with them to a visit.
Questions regarding how and when to use voice technology to best effect while respecting patient privacy are also at the fore of conversations around implementing MVT on broader scales.
Precision and recall for medical terms are fairly high in some emerging voice technologies.
In speech recognition, precision is about the validity of what’s captured and recall is about how completely the conversation was captured. The higher the rates of precision and recall, the more likely it is that what is transcribed will be relevant to effective and personalized patient care.
In a Google- and LinkedIn-sponsored study on speech recognition for medical conversations, precision for one MVT model studied was 92 percent, and recall was 86 percent when recognizing important medical phrases. The other MVT model studied reached 92 percent recall in reference to drug names used for treatment. Both technologies still required data clean up by clinical documentation specialists or providers.
The Limitations of Medical Voice Technology
Capturing conversations clearly is a challenge.
In digital medical scribe development, researchers assert that high-quality audio is essential to minimize errors in the processing pipeline. High-quality audio requires high-quality microphones, the patient and the provider in adequate proximity to the microphone, and as little background noise as possible.
While this may be simple to implement in provider offices, this need may pose a much greater challenge in a hospital or other open-floor plan setups. High-quality technology also brings up issues of equity as well. If MVT becomes universalized, will providers in facilities serving the economically marginalized get set up with the needed technology to bring forth the full promise of MVT? Or will the medical errors associated with low-quality audio recordings or inadequate recording setups become a part of what keeps inequality raging across our healthcare system?
Conversational speech doesn’t follow structured rules.
Humans don’t converse in a way that follows logical rules or patterns. People start sentences in one way and end in a way that is completely unrelated, add in unneeded filler words, repeat themselves, use discourse markers and filler phrases, interrupt one another, mumble, speak with different accents, and talk simultaneously.
In the Google/LinkedIn study, these circumstances led to less-than-ideal outcomes. For example, when utterances are unintelligible, human transcribers can use context clues to fill in what was said. MVT programs deleted whatever wasn’t understood. When people talked over each other, one of the MVT models deleted words in that region of audio. While precision and recall were high for medical phrases, the models both struggled to accurately capture casual conversation. In addition, one MVT model replaced things said in casual conversation with medical terminology that was not uttered.
The current word error rate in automatic speech recognition engines is 50 percent.
Despite the promise emerging in the Google/LinkedIn study, a 2018 study comparing the word error rate for existing automatic speech recognition (ASR) engines is not as rosy. The average word error rate during clinical conversation was 50 percent, with a range of 35 to 86 percent. As researchers point out, errors of this rate can really impact confidence in the transcription.
The Bottom Line: Medical Voice Technology
While the promise of what MVT can do is vast, the current reality is that humans are still needed in a major way to ensure the accuracy of what is captured. Right now, MVT can’t yet handle the realities of bustling medical environments, the spontaneous nature of human conversation, or the equitable technological distribution needed to deliver providers with freedom, and clinical medical records with accurate inputs.
The Benefits and Promises of Using AI for Clinical Documentation
In a world where medical voice technology is 100 percent accurate, AI transforms what medical voice technology captures into clean documentation ready for sharing and billing, a patient-centric medical diagnosis, and treatment plan recommendations based on vast amounts of data. All of this can lead to fewer mistakes, more productive providers, and happier, healthier patients.
Privacy is embedded in conversations about AI development.
As mentioned earlier, the legacy of HIPAA in healthcare means those who are developing medical AI understand that privacy must be intrinsic to design. For example, The Primary Care Informatics Working Group of the International Medical Informatics Association has a series of recommendations for the ethical use of AI in clinical documentation that include formal processes for consent and access to data, sustainable data creation, and collection. They emphasize maintaining trust and permission, paying attention to the risks inherent to de-anonymizing of data that can occur in AI mediated systems, as well as a recognition that ethical issues will need to be addressed on an ongoing basis.
Those studying ethics in a world with AI also believe that privacy can be protected through legislation that pushes for transparent AI systems, deeply rooted rights to collect information, opt-out rights, limitations on data collection, and the capacity for patients to delete data upon request.
AI is successfully being used in some realms of medicine.
Forty-four percent of healthcare organizations use AI already, and some to great effect. In the world of imaging, for example, AI can diagnose skin cancer better than board-certified dermatologists, and algorithms are assisting radiologists in making accurate breast cancer diagnoses by providing second opinions on mammograms. The promise of AI is being realized, and with enough development, perhaps that success can translate into the world of documentation.
The Limitations of AI in Clinical Documentation
The Extract-Transform-Load (ETL) process may reverse efforts to protect privacy and destroy data accuracy.
In order for there to be an ethical repository of medical data for AI to learn from, data from clinical documentation needs to be sent anonymously to a data warehouse. For all AI to be able to learn from the data, it needs to be formatted in a way that other AI can understand and relay to medical providers. This data warehousing is currently accomplished through a process called Extract-Transform-Load (ETL).
During the ETL process, data is extracted from the data source, and transformed to fit the schema of the communal data warehouse in a staging area. Once transformed so that it can be universally understood by other AI, the “clean” data is loaded into the data warehouse.
One issue with the ETL process is that it puts patient data at risk of re-identification. According to one study, large machine-learning algorithms can re-identify a record utilizing as few as three data points. This further complicates the currently inadequate patient consent and notification regarding data use. Essentially, the use of AI for clinical documentation currently has the power to nullify the patient’s right to privacy.
Another struggle inherent to the ETL process is that accuracy of information can get lost when the data is being transformed for storage in the warehouse. Accuracy problems have downstream impacts that can lead to improper diagnosis, missed calls, and other mismanagement of patient cases that have real-life negative impacts on patients and medical providers.
Some medical AI currently renders recommendations without rationale.
In the world of AI development, there is a term called “black box” deployments. In a black box deployment, medical AI analyzes the data at hand and shares its finding, diagnosis, and treatment recommendations but doesn’t provide the rationale or evidence for those decisions.
This lack of transparency can result in issues when a black box recommendation that is utilized doesn’t work, or when black box recommendations are making recommendations that don’t make sense. If humans can’t help the decision-making algorithm to adjust and adapt for accuracy, there’s no way to help AI adjust and adapt when needed.
Human-created documentation has ancillary benefits for the care delivered by medical providers.
While spending endless hours clicking through checklists is not the best way for a provider to use their time, completely transferring documentation burden to AI may have unintended consequences for patient care.
There may be some benefits to writing patient documentation by hand. According to research, the act of writing clinical narratives improves observation, helps providers to cultivate empathy for patients, and engages critical thinking. In addition, the narratives written by providers include things that AI can’t pick up on like non-verbal cues, innuendo, social cues, and more.
AI are human creations that can take on human bias.
Despite AI not being human, the machine learning behind some AI is modeled by humans, and AI utilizes human-generated data to discern how to complete the clinical documentation it then references to make recommendations that impact humans.
According to a paper published in the AMA Journal of Ethics, the potential of AI only extends as far as the dataset it is accessing. If the data only reflects individuals who have access to healthcare, or only represents findings on certain demographic groups, then the AI analyzing that data can invisibly and unintentionally reproduce bias.
Another AMA Journal of Ethics study found that AI predictions led to bias in outcomes for ICU mortality based on gender and insurance type, for example. Because AI is not advanced enough to discern and eliminate bias, it’s possible that AI can actually exacerbate the health disparities that already exist.
Competition is getting in the way of the quality and equity AI could bring to medicine through clinical documentation.
According to healthcare information management scholars, substantial amounts of data are needed for medical AI to perform at its highest potential. This requires millions of observations before rendering recommendations of true quality.
Obtaining and sharing medical data is complex in a world divided by borders and consumed by the monetization of data. Compounding that complexity is the fact that EHRs were developed in a competitive market, which stymied cooperation, led to massive barriers to interoperability, and resulted in a heterogeneous dataset that can’t be reconciled without losses to medical accuracy or countless hours of human intervention.
While properly anonymized medical data could be shared freely between our medical establishments and across our planet to improve outcomes for all, competition and monetization serve as barriers to that ever happening.
The Future of Voice Technology and AI for Clinical Documentation
The dream of how voice technology paired with AI could revolutionize the role of clinical documentation in medicine is currently just that—a hazy vision of how things could be in the future.
The current reality is that data privacy is a concern but not easy to implement; market competition and nationalism isolate our knowledge bases and bar us from creating the universal compendium of knowledge that would benefit the AI mediated documentation process (and us all); and voice-to-text technology is factually not sophisticated enough for use in most medical environments.
While patients, medical providers, and clinical documentation specialists alike would most definitely benefit from this combination of technology, making it real, accessible, and equitable will be a challenge that requires cooperation on scales never before seen across medicine, business, and nations.
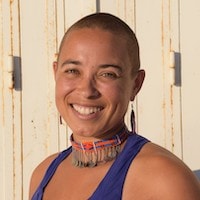
Becca Brewer, MEd
WriterBecca Brewer is building a better future on a thriving earth by healing herself into wholeness, divesting from separation, and walking the path of the loving heart. Previously to her journey as an adventurer for a just, meaningful, and regenerative world, Becca was a formally trained sexuality educator with a master of education.